DEEPScreen: high performance drug-target interaction prediction with convolutional neural networks using 2-D structural compound representations
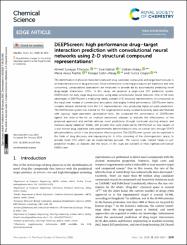
View/ Open
Date
2020Author
Rifaioğlu, Ahmet SüreyyaNalbat, Esra
Atalay, Volkan
Martin, Maria Jesus
Çetin-Atalay, Rengül
Doğan, Tunca
Metadata
Show full item recordCitation
Rifaioglu, A.S., Nalbat, E., Atalay, V., Martin, M.J., Cetin-Atalay, R., Doǧan, T. (2020). DEEPScreen: high performance drug-target interaction prediction with convolutional neural networks using 2-D structural compound representations. Chemical Science, 11 (9), pp. 2531-2557. https://doi.org/10.1039/c9sc03414eAbstract
The identification of physical interactions between drug candidate compounds and target biomolecules is an important process in drug discovery. Since conventional screening procedures are expensive and time consuming, computational approaches are employed to provide aid by automatically predicting novel drug-target interactions (DTIs). In this study, we propose a large-scale DTI prediction system, DEEPScreen, for early stage drug discovery, using deep convolutional neural networks. One of the main advantages of DEEPScreen is employing readily available 2-D structural representations of compounds at the input level instead of conventional descriptors that display limited performance. DEEPScreen learns complex features inherently from the 2-D representations, thus producing highly accurate predictions. The DEEPScreen system was trained for 704 target proteins (using curated bioactivity data) and finalized with rigorous hyper-parameter optimization tests. We compared the performance of DEEPScreen against the state-of-the-art on multiple benchmark datasets to indicate the effectiveness of the proposed approach and verified selected novel predictions through molecular docking analysis and literature-based validation. Finally, JAK proteins that were predicted by DEEPScreen as new targets of a well-known drug cladribine were experimentally demonstrated in vitro on cancer cells through STAT3 phosphorylation, which is the downstream effector protein. The DEEPScreen system can be exploited in the fields of drug discovery and repurposing for in silico screening of the chemogenomic space, to provide novel DTIs which can be experimentally pursued. The source code, trained "ready-to-use" prediction models, all datasets and the results of this study are available at ; https://github.com/cansyl/DEEPscreen.